Data analysis is a critical component of scientific research, helping to extract meaningful insights from raw information. Proper techniques ensure that findings are accurate, reliable, and valuable for future studies. If you’re working on scientific research, following best practices for data analysis in science is essential. Here, we’ll explore these practices to help you better manage your data.
1. Clearly Define Your Research Questions
Before you dive into any analysis, it’s important to define your research question. This step gives you a clear direction, helping you understand what you want to achieve through your data analysis. The data you collect should directly align with the research question, so there’s no confusion when interpreting results.
Why It Matters
By having a clearly defined research question, you avoid gathering irrelevant data and focus on the information that will lead to meaningful outcomes. This saves time and enhances the efficiency of the entire research process.
2. Collect High-Quality Data
Accurate and relevant data is the foundation of sound scientific analysis. Ensuring the quality of the data you collect is crucial for achieving valid results. It’s essential to minimize biases and errors during data collection, as these can affect the validity of your findings. Make sure your data is reliable, whether you’re gathering it from experiments, surveys, or other methods.
Why It Matters
Poor-quality data can lead to incorrect conclusions, reducing the reliability of your research. High-quality data, on the other hand, provides a strong base for accurate analysis and credible scientific discoveries.
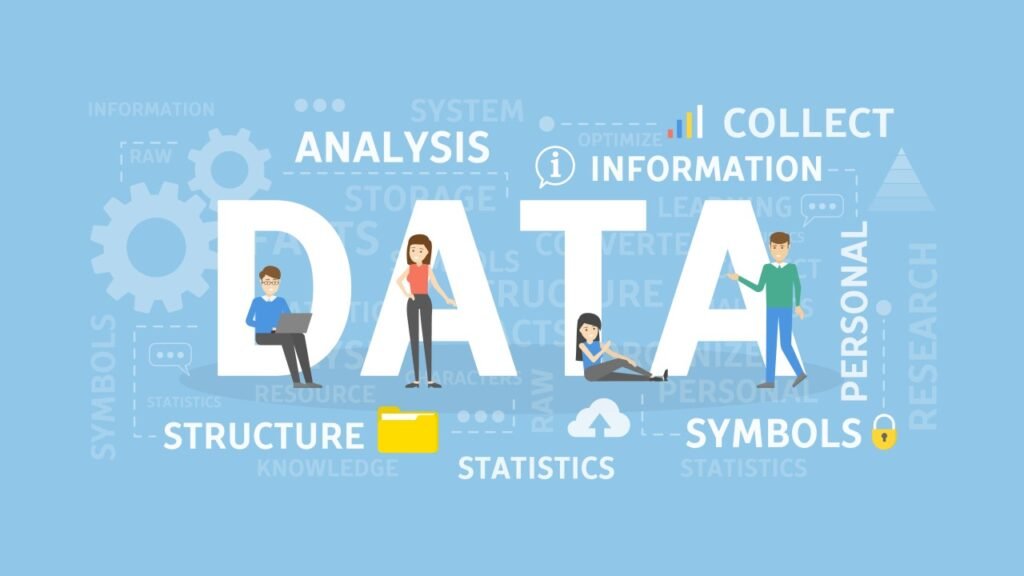
3. Use the Right Tools and Software
In today’s scientific landscape, numerous software tools are available for effective data analysis in science. From SPSS and R to Python and Excel, choosing the right tool depends on the complexity of your data and the type of analysis you need to perform. These tools can help you process large datasets efficiently, run statistical tests, and visualize your findings.
Why It Matters
Using the right tools ensures that your analysis is conducted efficiently and accurately. Different types of research may require different tools, so selecting the appropriate software can enhance the accuracy of your results.
4. Ensure Proper Data Cleaning and Preparation
Before starting your actual analysis, cleaning and preparing your data is a must. This process involves eliminating outliers, filling in missing values, and correcting any inconsistencies within the dataset. Clean data minimizes errors and improves the clarity of your results.
Why It Matters
Unclean data leads to inaccurate analysis and misleading conclusions. Data preparation sets the stage for successful analysis, ensuring that the data is ready for deeper interpretation.
5. Choose the Right Statistical Methods
When analyzing scientific data, selecting the appropriate statistical method is key. Depending on your research objectives, you might use techniques such as regression analysis, ANOVA, or chi-square tests. Applying the right statistical method allows you to identify patterns, relationships, and insights from the data.
Why It Matters
Choosing the wrong statistical method can produce inaccurate results, skewing the interpretation of your data. Using the correct method ensures that the insights drawn are reliable and valid.
6. Interpret Results Objectively
Objectivity is critical when interpreting your data. While it can be tempting to find results that support your hypothesis, maintaining neutrality allows for honest conclusions. Pay attention to both expected and unexpected outcomes, as they can provide valuable insights that contribute to scientific knowledge.
Why It Matters
Objective interpretation reduces the likelihood of biases influencing the results. By considering all possibilities, you make your analysis more credible and allow for a broader understanding of the data.
7. Visualize Data for Better Understanding
Visual representation of data helps make complex results easier to understand. Using charts, graphs, and tables can enhance the communication of your findings. Visual tools such as scatter plots, bar graphs, and heat maps allow both researchers and non-experts to grasp your results quickly and effectively.
Why It Matters
Data visualization bridges the gap between numbers and comprehension, making it easier to interpret and communicate your findings. It also provides a clear way to showcase key trends and insights in your research.
8. Document and Share Your Process
Maintaining clear documentation of your analysis process is essential. This includes recording the steps you took, the tools you used, and the rationale behind your decisions. Sharing this information makes it easier for others to replicate your research, improving the transparency and credibility of your work.
Why It Matters
Documentation fosters collaboration and transparency in the scientific community. Sharing your methods ensures that other researchers can replicate your findings, adding to the collective knowledge in the field.
Conclusion
By following best practices for data analysis in science, you ensure that your research is accurate, reliable, and contributes meaningfully to the scientific community. From collecting high-quality data to interpreting results objectively and using the right tools, these strategies are essential for deriving sound conclusions. Remember, each step of the analysis process plays a role in shaping the credibility of your scientific findings, making it important to be thorough and objective.